Autonomous Home Living
Instructor: Marcelo Spina, Casey Rehm
Assistant tutor: Laure Michelon
Partner: Evelyn Junco
Site: El Segundo, CA
Rapid advances in artificial intelligence are reshaping our way of living, working and socializing. We rely on the role of automation to quickly generate and explore alternatives of our present reality.
Automated vehicles technology has raised issues of relationship between human, streets and living spaces. Moreover, on a rapid increase of traffic congestion and a new generation changing home ownership habits; the project proposes housing for a community that strives for a balance between temporary enclosed spaces and ridesharing.
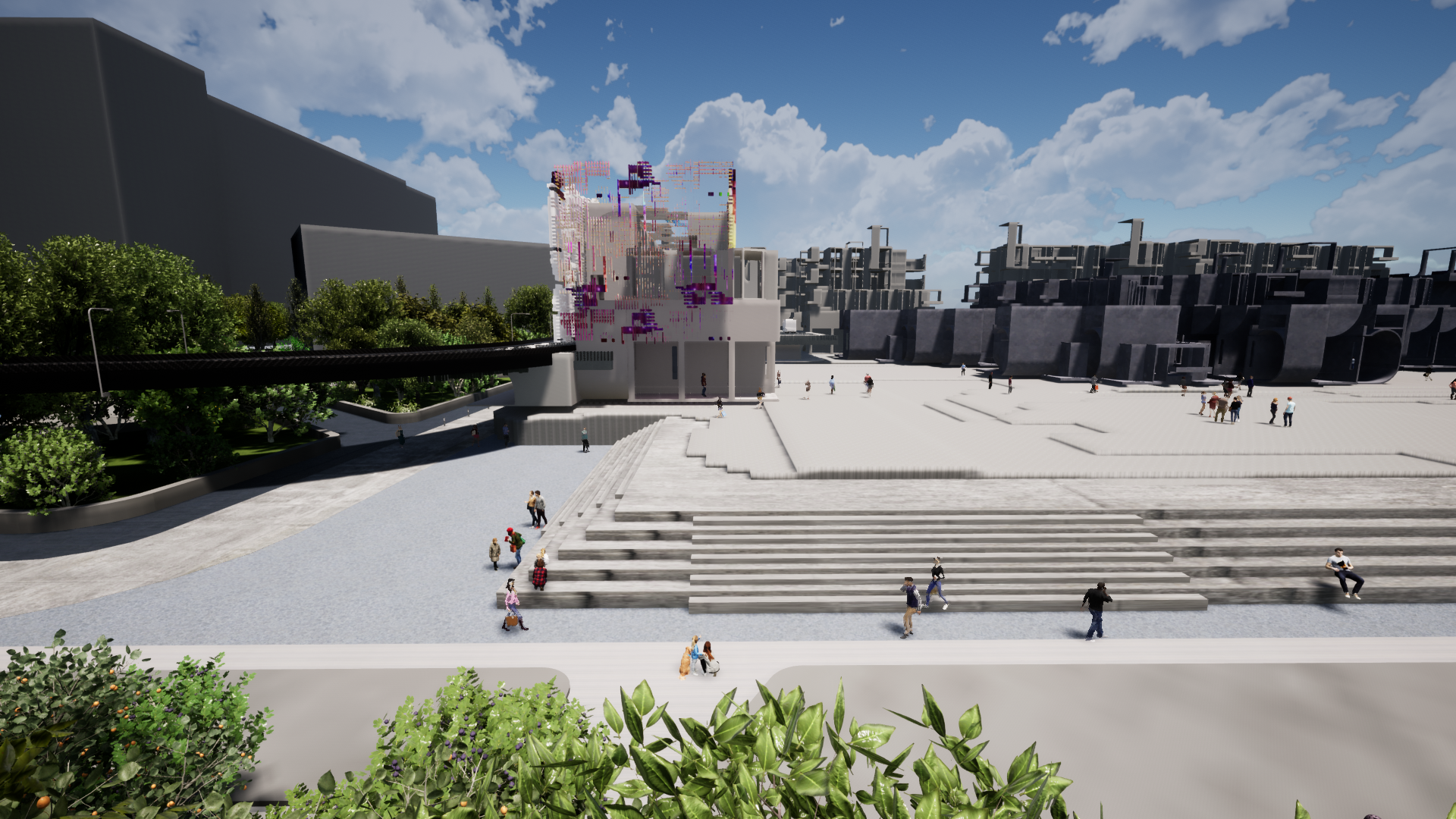
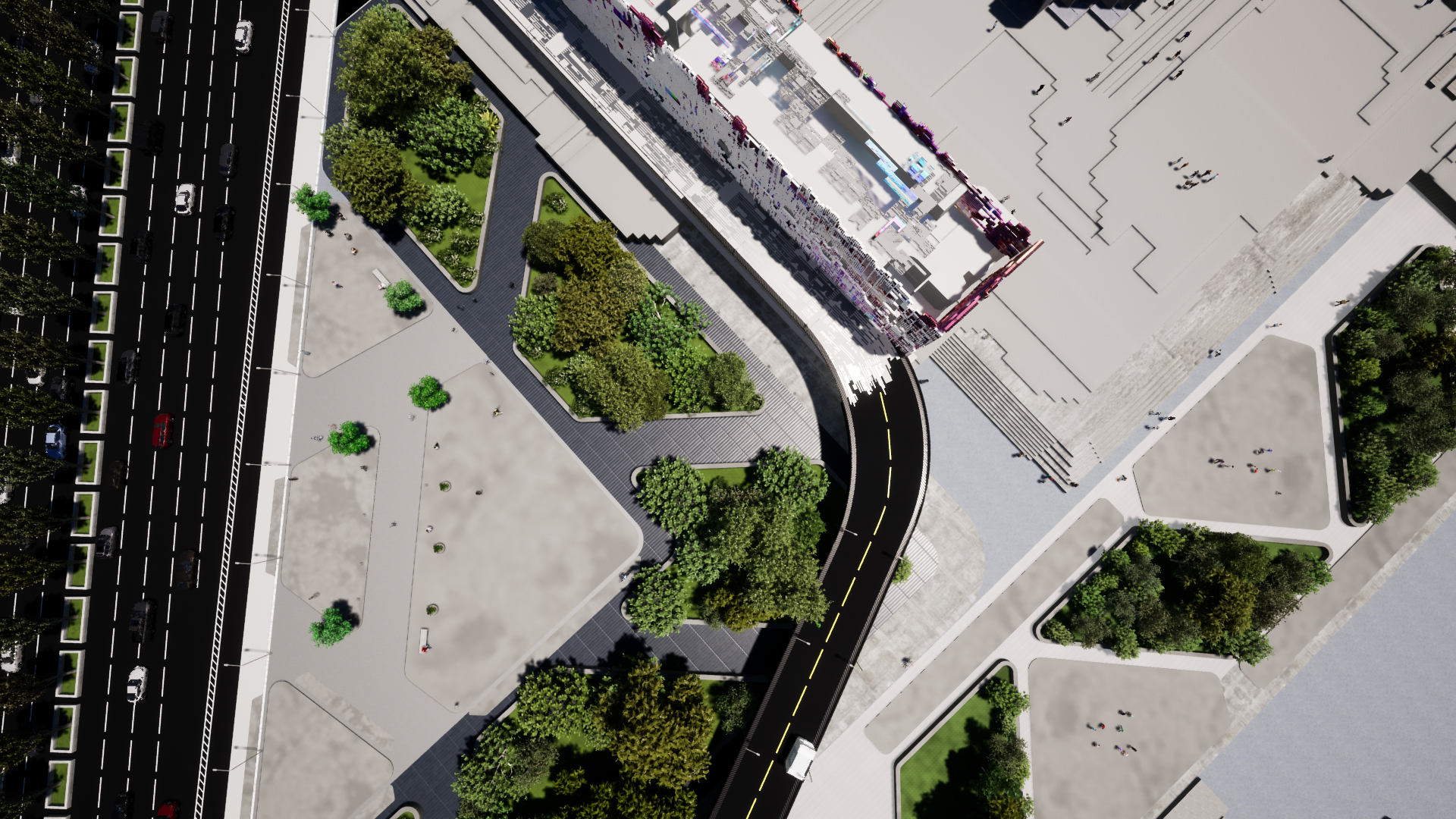
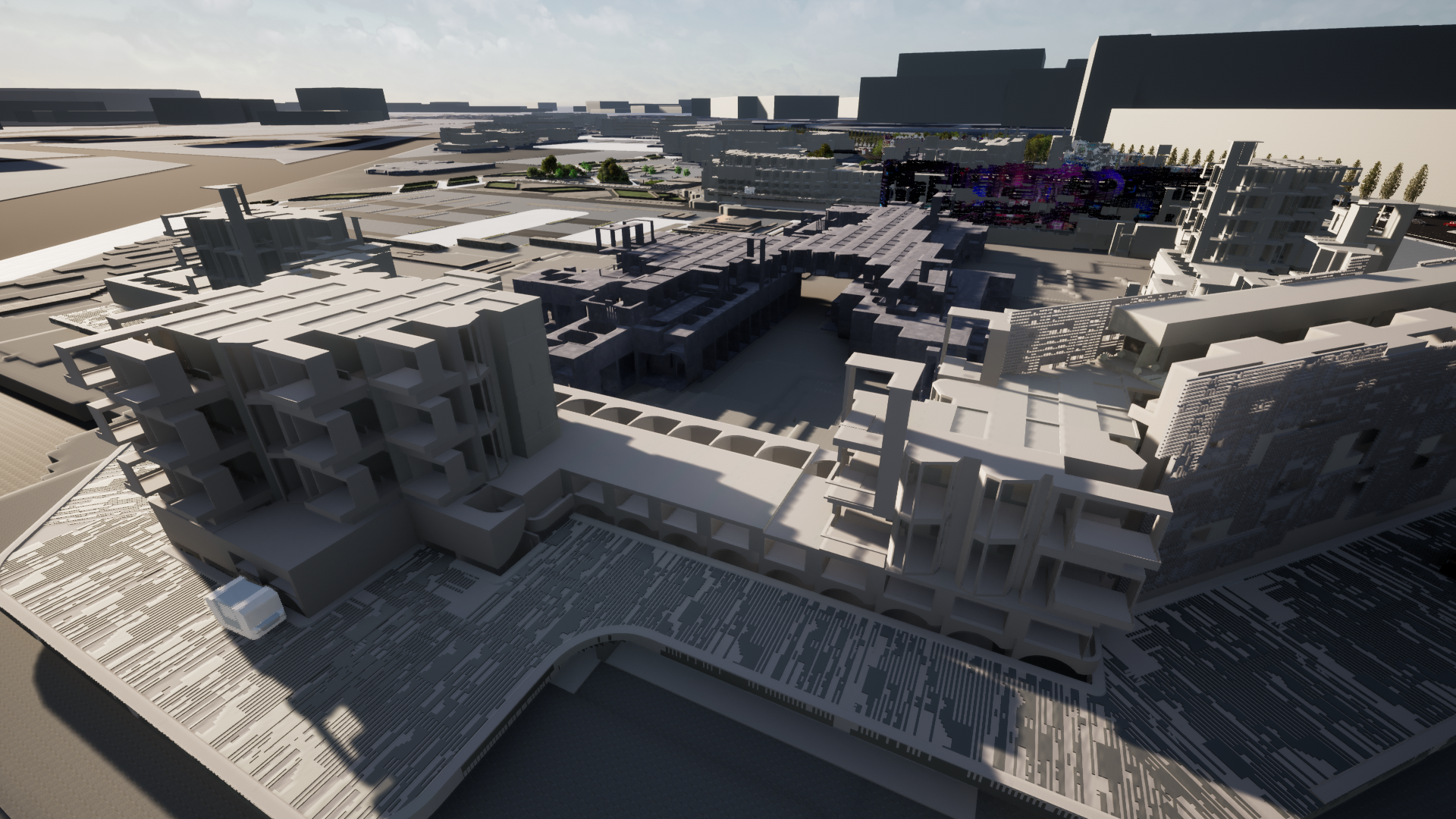
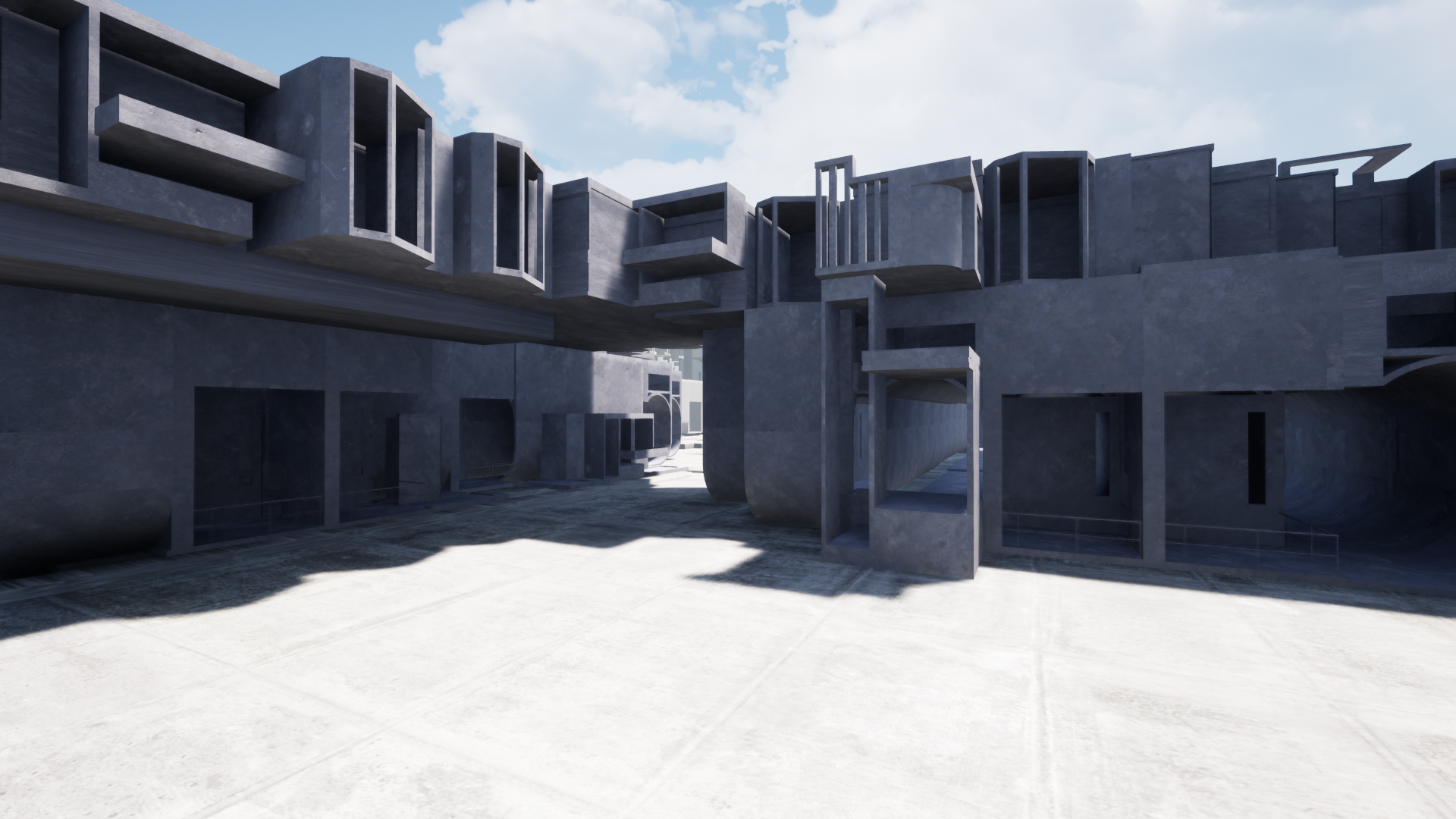
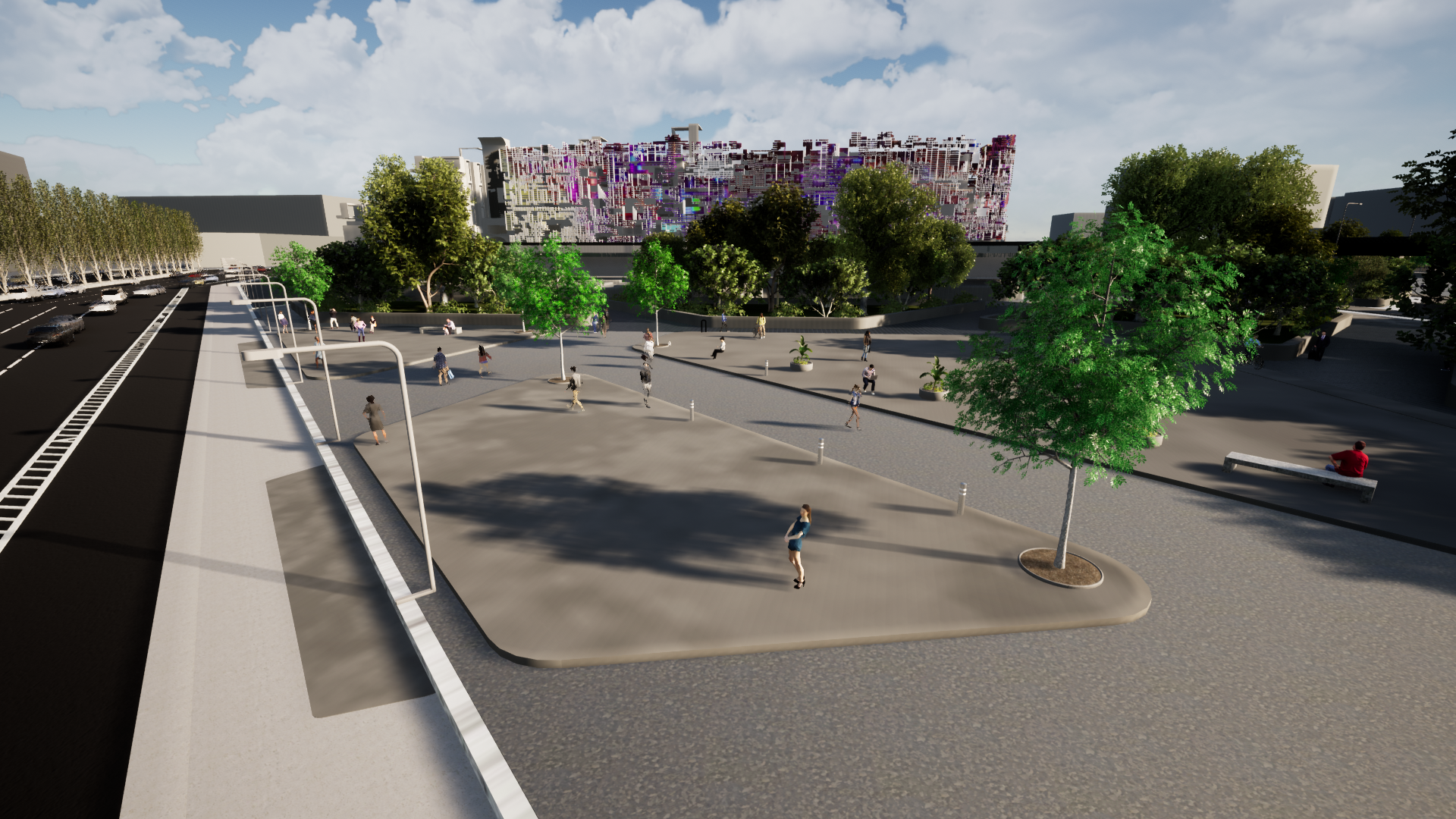
Concept
Automotive industries are exploring the idea of a vehicle that can be attached and detached from a living space. The autonomous home living could radically reduce carbon footprints and living expenses by combining transportation and housing needs in one space.
New types of communities will emerge reshaping our streets and public spaces. A car receives an order that will be cooking on the road; or automatically selects items to deliver.
Our speculation extends to the idea that the building envelope is not only designed for humans but also for machine vision.
Automotive industries are exploring the idea of a vehicle that can be attached and detached from a living space. The autonomous home living could radically reduce carbon footprints and living expenses by combining transportation and housing needs in one space.
New types of communities will emerge reshaping our streets and public spaces. A car receives an order that will be cooking on the road; or automatically selects items to deliver.
Our speculation extends to the idea that the building envelope is not only designed for humans but also for machine vision.
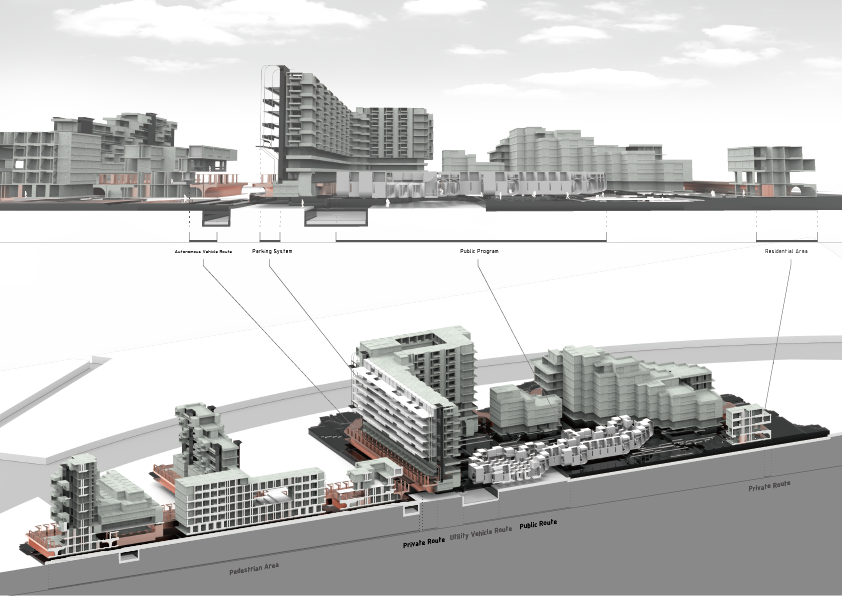
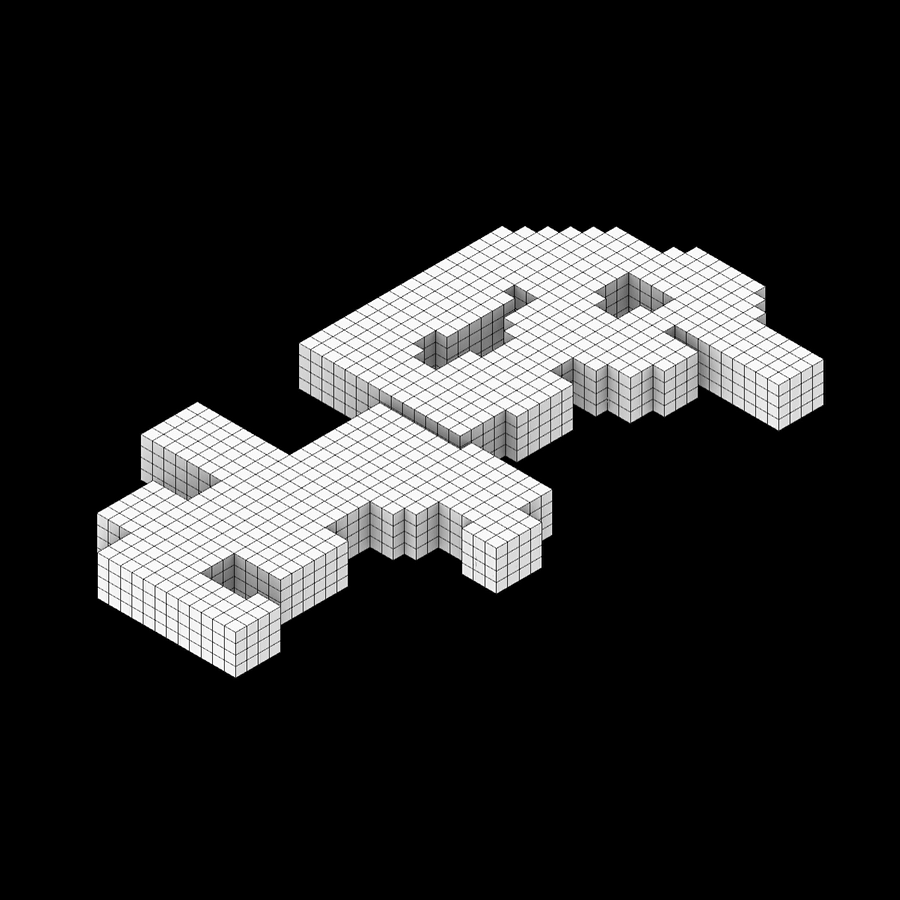
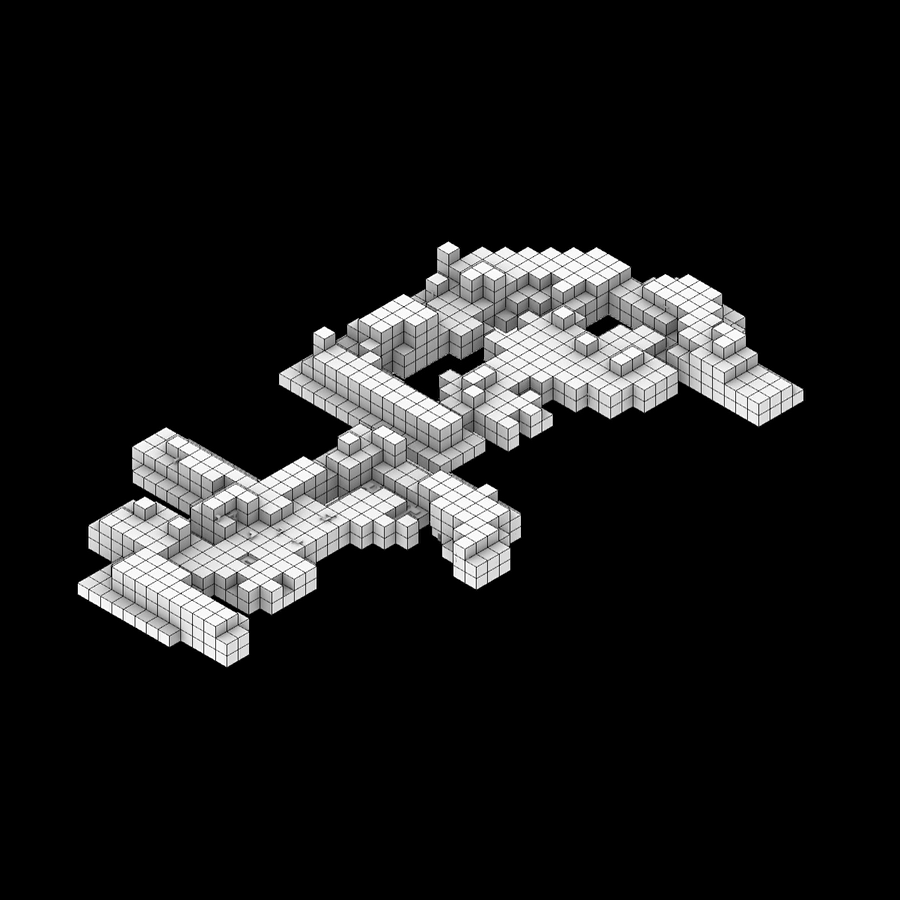
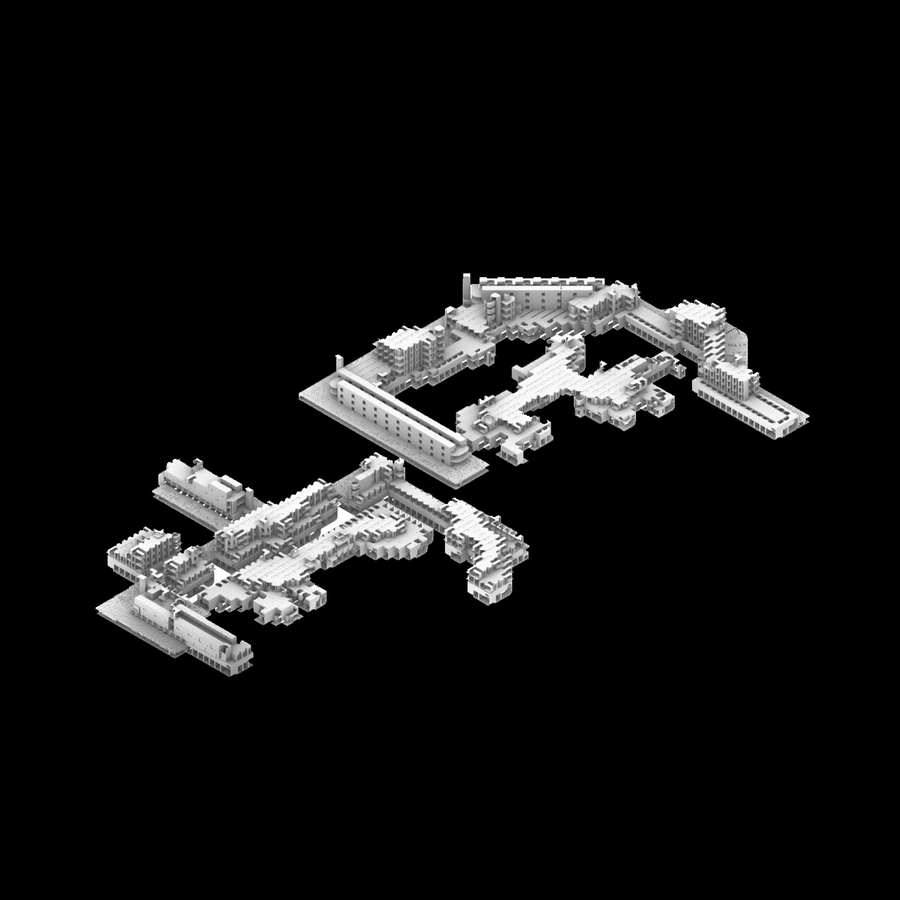

UrbanPlan
The urban design of our site, located at El Segundo, was a process of generative deep learning. Using cyclegan, an image-to-image translation technique, we processed existing urban plans as our input, and in return, the machine generated a new urban fabric for the entire site. Our design concentrates and takes a closer look at these two masses as a proof of concept for a large masterplan.
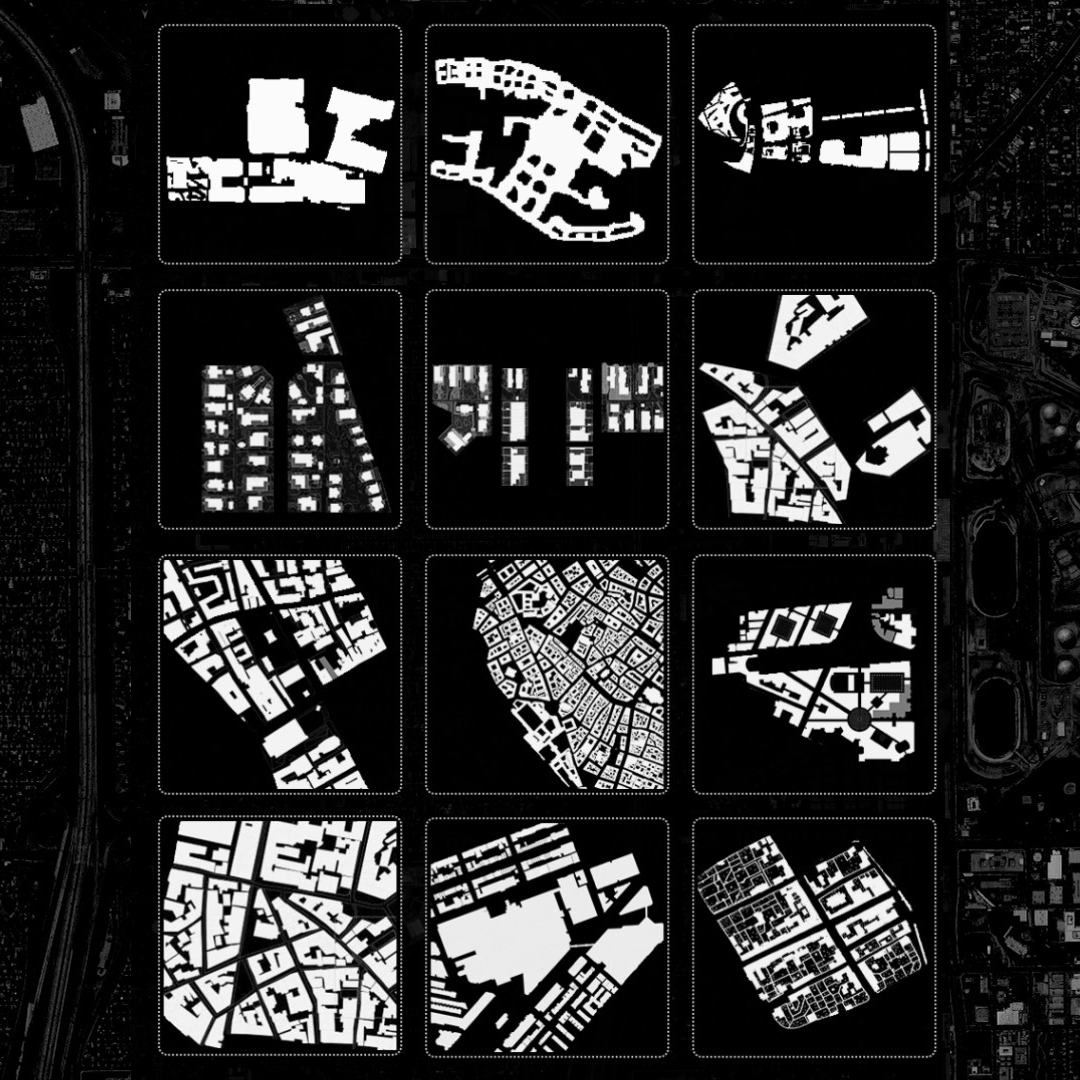
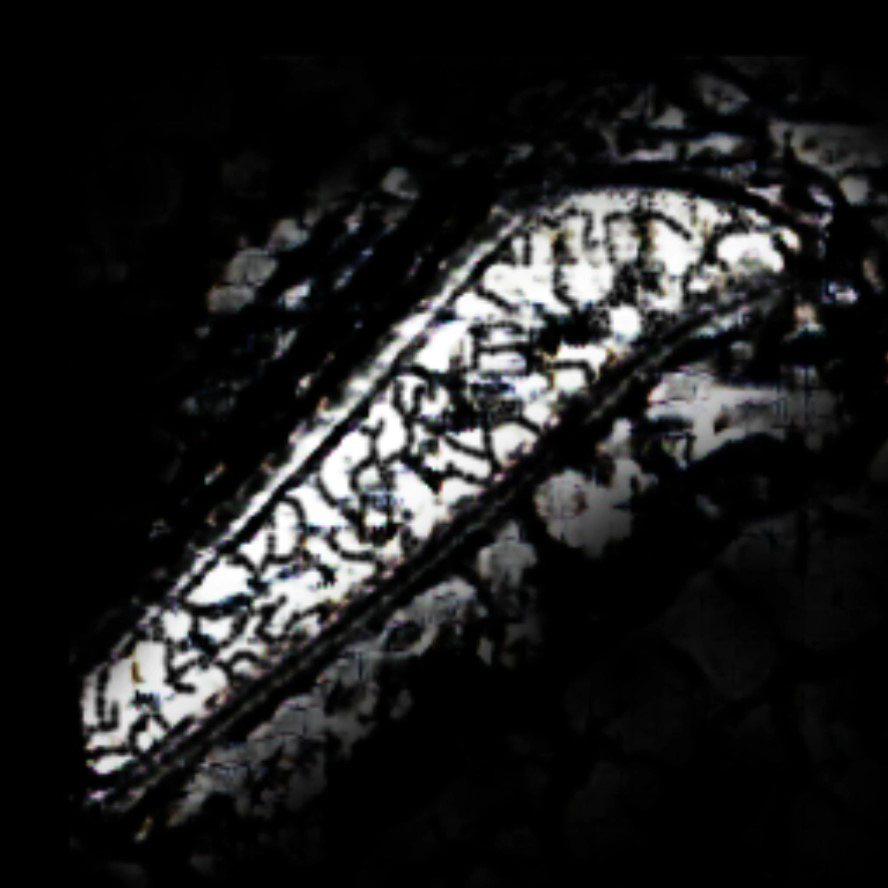
Building Assembly
The building assembly was thought to define public vs private spaces, where a route serving autonomous cars will tie the masses to the site. The car route carves into the masses to create pockets of shared communal areas. Its massive composition of modular elements and imposing geometric lines became the influential element on the design of the project.
Starting from the idea of repetitive housing modules, the aggregation of 4x4 modules creates interior spaces, terraces and shared spaces. The same source produces different outcomes and that will ultimately be fused back into one and through the design of the building.
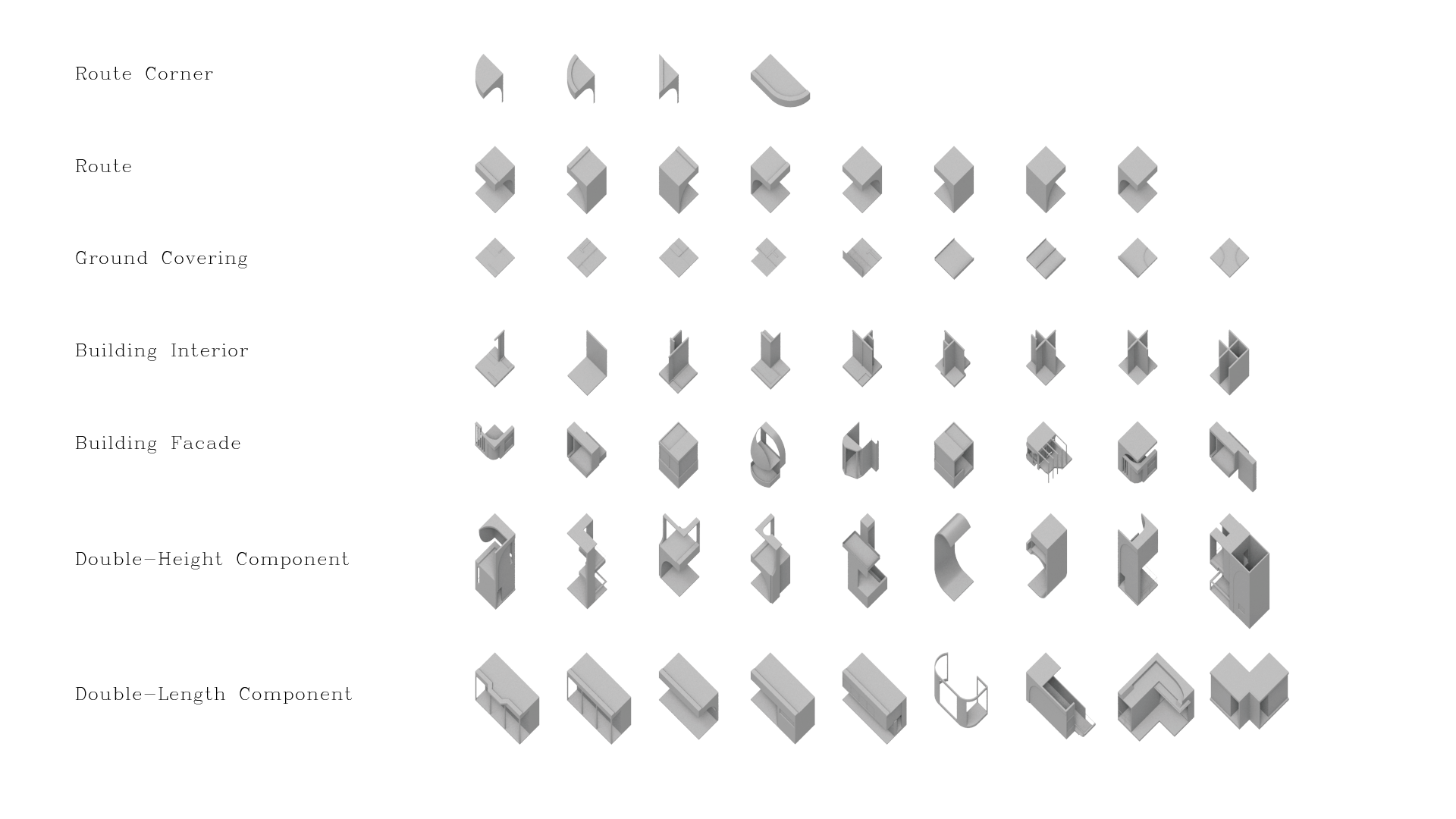
Facade
The facade is a dynamic three dimensional ever changing texture built using the method of degenerative design. Several trained images by cycleGAN are projected into a repetitive movable pattern. The abstract patterns and shifting pieces of the structure explore the transformation of visible and invisible elements.
By doing so, it allows different patterns to be visible to the autonomous car but invisible to the human eye. Then, the user is able to choose within available units. The vehicle can stay parked as part of the housing unit or drop the users off.
Taking a closer look at this movable pattern. The car attaches to the unit, the interior door closes and the car is locked to the facade pattern. Most important, this pattern allows the vehicle to charge while it is parked. The self-driving vehicles can adapt to diverse functions making the residential units purposely flexible. They can function as an office space or start-up business since it is the movable part of the house.
Object Detection
Looking at the facade from the car's perspective, this is able to detect which units are available. Object detection often requires vast datasets and long training times. To make this project possible, we applied two simplifications: we trained and labeled the renders of our own geometry and we predicted a fixed number of objects in each image.
Yolov4
Most modern models require many GPU for training with a large batch size, which makes training really slow. Yolo is an object detector model which can be trained on a single GPU with a smaller batch of images.
Since our machines were learning our own facade design and patterns, which were digitally produced and not real objects, we needed to label the position of each pattern and input several renders of the same pattern at a different angles and different backgrounds.